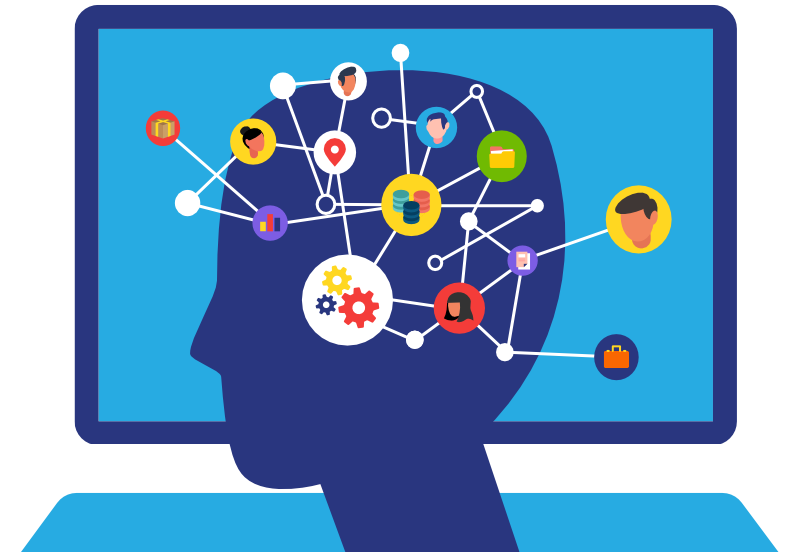
The world is moving at a faster pace, and new technologies are coming up to shape the industries and the entire globe. This will traverse the whole universe, from Africa to America, Europe to Asia. One of these emerging technologies is Artificial Intelligence, also known as AI. Artificial Intelligence has gained momentum in the world like other technologies such as Internet of Things (IoT), Blockchain technology, 3D printing, robotics, etc.
Meanwhile, as great as the promise of AI is, it depends on certain ingredients for successful adoption; one of the critical ingredients for a successful AI implementation is clean, representative and large amount of historical data. However, unless you are Google or Facebook or handful of other digital native companies blessed with vast amounts of representative data, you will struggle to harvest historical data that are sufficiently representative enough or in the right form to give the required inference for machine learning techniques to be an effective enabler for AI initiatives. Therefore, to make AI work, there is a need for an improved level of data preparation, engineering, enrichment, and contextualization. One successful approach to solving this conundrum is by harnessing the power of AI without the need for large amounts of representative historical data; to find out how to do it, sit back, take a cup of coffee or tea and read further.
Data: A Reservoir of ‘potential’ Knowledge
Data is the single most important component in the life of Artificial Intelligence. Without data, AI cannot function. For example, let’s take self-driving cars which is probably the most popular application of Artificial Intelligence. To build a self-driving car, huge amount of data is needed; this includes images from digital cameras as well as signals from infrared sensors and high-resolution maps. Research shows that self-driving cars produce 1 terabyte of raw data per hour. Thereafter, all the raw data is used in developing AI models that drive the car and over a period of time with more data being fed to the machine, it will get better at doing the task it was meant to do. A key point to note here is that the machine is looking for patterns from vast amounts of ‘dumb’ data and the machine’s ability to recognize these patterns at the quickest possible time with highest level of accuracy makes all the difference as opposed to having ‘smart’ data to infer from.
From Data to Knowledge (intelligent data) through Semantic
Enterprises are inundated with silo IT systems built over the years that contains data designed to do very specific individual ‘System of Record’ tasks; but unfortunately these records are duplicated across multiple ‘Systems of Record’ resulting in massive data proliferation but lacking complete representation of an entity in any single system. For example, if a business user wants to know relevant information about her customer, she will need to access the corporate CRM system to learn about previous customer interactions; if the user wants to know about her customer’s previous purchase behavior, she may need to access a Sales Transaction & accounting systems; if she wants to know if there has been any complaints from this customer, she may need to access yet another Support and Feedback management system and all this while hoping that a universal customer identifier has been used across all these myriad of systems. This reality has given rise to fragmented and often duplicated data landscape that requires expensive and often non-efficient means of establishing ‘Source of Truth’ data sets.
The semantic graph model involves the process of structuring data to store it in a clear and logical way – through a graph with connections, just like how our brain stores information. It adds meaning to the data as well as the relationships between them. Semantic graph by its very nature is flexible and malleable; depending on one’s desired outcomes, one can get started with a schema-less frame-work and build one’s way up to complex structures that enable reasoning, inferencing and knowledge engineering activities.
Intelligent Data gives Intelligence to AI
AI applications improve as they gain more experience and contemporary AI applications have an unhealthy obsession with gaining this experience exclusively from Machine Learning techniques. While there is nothing inherently wrong with Machine Learning, the main caveat for a successful Machine Learning outcome is sufficient and representative historical data for the machine to learn from. For example, if an AI model is learning to recognize chairs and has only seen standard dining chairs that have four legs, the model may believe that chairs are only defined by four legs. This means if the model is shown to, say, a desk chair that has one pillar, it will not recognize it as a chair. This gives a flavor of the gargantuan task of even coming to terms with “what exactly is a representative data set that has enough nuances that is unique to my problem from which the machine can detect the patterns and learn from it” and we have not even talked about “how much data is sufficient?”
This is where an intelligent data represented by semantic graph accelerates an AI application development by starting with a deterministic semantic graph where entities are connected based on real-world assertions. For example a customer graph may contain facts such as, ‘customers’ are a type of ‘person’; ‘person’ has ‘name’, ‘date of birth’ and ‘email ID’; same person does not have 2 distinct ‘dates of birth’; ‘customers’ buy ‘products’ from ‘organizations’; and the entities and their connections goes on and on. By starting with a deterministic model, an organization can fast track its AI implementation activity and then employ Machine Learning techniques to augment the semantic graph model as a steady stream of representative data sets are fed into the machine. From our experience, this approach has proven to be a far more sustainable approach (and produces faster business outcomes) in comparison to struggling with ‘cold start’ problems associated with Machine Learning techniques.
Getting Started with Artificial Intelligence
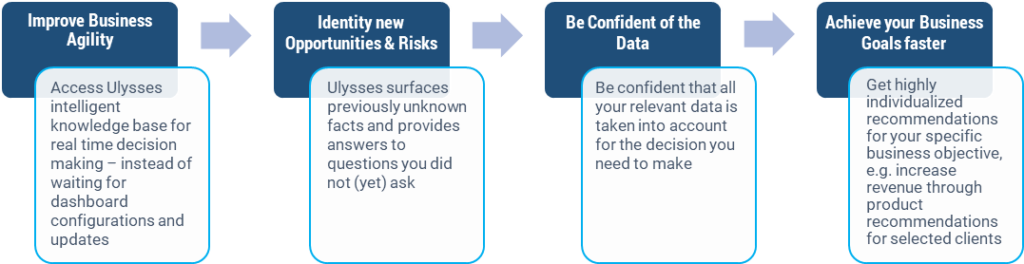
Benefits of Latize Ulysses
At Latize, we understand the importance of the semantic graph model. Therefore, all our solutions are built around this model simply to help your company grow and achieve its corporate objectives. Many companies are faced with the challenge of either struggling with what they can do with the raw data they generate from their business operations and/or ‘how do we show value out of our AI initiatives in the quickest possible time?’. With Latize, over the years we have figured out a way to put the intelligence back in data so that our customers can get quantifiable outcomes from their AI initiatives in the quickest time possible. That’s what we do and we do it best. Get in touch with us today to find out how we can make your data work for you, more intelligently.